8 Steps to Ensure Robust Data Quality Assurance for Your Business
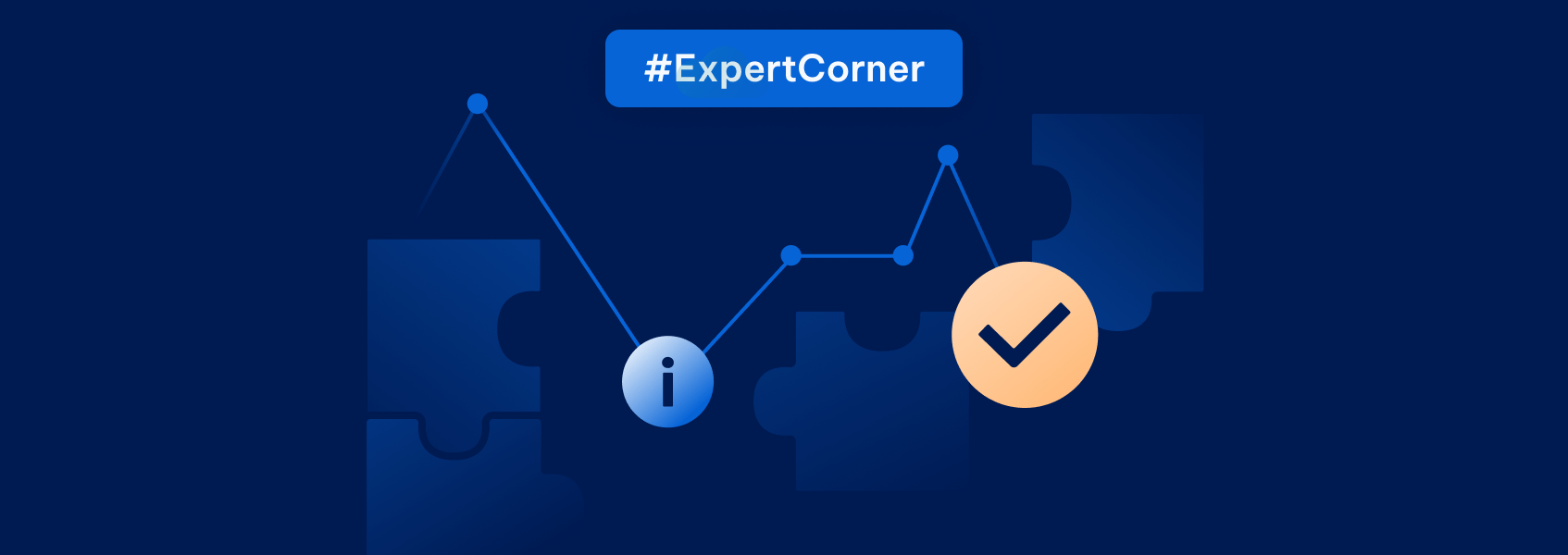
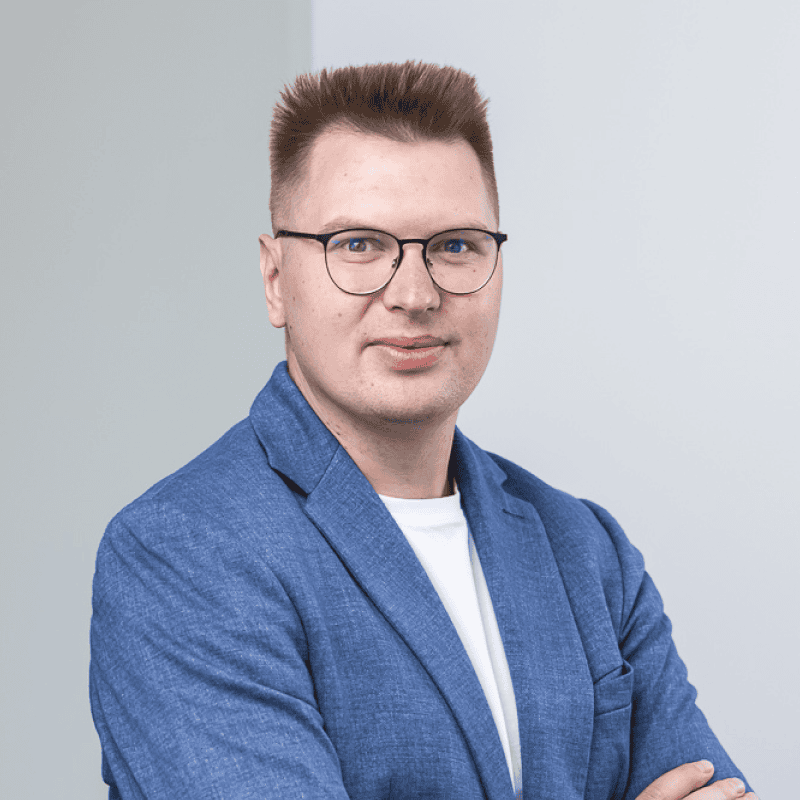
Justas Vitaitis
Last updated -
In This Article
Despite having access to large amounts of data, some businesses struggle to reap its benefits. There are several reasons for this, but one main one is data quality. The quality of the data you use impacts your outcomes.
Quality data helps you understand and improve business processes so you can reduce wasted money and time. Poor-quality data does the opposite. It can lead to inefficient processes, wasted resources, and missed opportunities — that’s definitely not something you want.
That’s why data quality assurance is important. It’s a proactive approach to ensure data is accurate, complete, consistent, and reliable for its intended use.
In this article, we’ll show you how to ensure robust data quality assurance for your business.
8 Steps to Implement Data Quality Assurance
According to research , businesses that invest in data and analytics report a profitability or performance increase of at least 11%.
Organizations that use reliable data make fewer errors, improve their processes, and, ultimately, make better decisions. Below are steps to ensure the data you rely on is accurate:
1. Define Data Quality Metrics
The first step toward ensuring data quality assurance is outlining what your organization considers “good” data. This is where defining data quality metrics comes into play.
Data quality metrics are standards used to assess the quality of a dataset. Choosing the right metrics and using them to assess data ensures an organization has the high-quality data it needs.
Data quality metrics include:
- Accuracy
- Completeness
- Consistency
- Timeliness
- Duplication
- Uniqueness
To choose the right ones, consider your specific organizational goals. Identify the key business processes connected to those goals that rely on data. Then, determine the impact of data quality on those processes and their outcomes. You will need to select the metrics that best evaluate this data.
Suppose your goal is to launch a product successfully. In that case, you may prioritize accuracy, completeness, and timeliness to ensure reliable decision-making through the product life cycle. However, if you intend to improve efficiency in your supply chain, duplication and consistency might be more critical for tracking inventory and shipment data.
You will also need to involve stakeholders and customers during this process. This ensures all relevant perspectives and requirements are considered when defining data quality standards.
Document all selected metrics, definitions, and acceptable thresholds in a comprehensive data quality framework. This will guide future data quality assessments and provide a reference point for maintaining consistent standards across the organization.
2. Discover Hidden Insights with Data Profiling
Once you’ve established the quality metrics, the next step is to profile your data. Data profiling helps you understand the distribution, patterns, and relationships within your dataset. As a result, you can identify potential issues, outliers, and inconsistencies that might lead to poor data quality .
Assume you’re analyzing customer contact information. Data profiling might reveal missing phone numbers, invalid email formats, or duplicate entries that need to be addressed.
This, then, informs the creation of targeted data cleansing processes (more on these later), ultimately leading to more accurate and reliable data.
You can use various techniques to carry out data profiling. Here are some of them:
- Column profiling
This data profiling technique leverages frequency analysis. It counts how often a value appears in a column. It then uses this information to detect patterns and generate statistics.
- Cross-column profiling
Cross-column profiling focuses on key analysis and dependency analysis. Key analysis identifies the most important columns in a dataset, while dependency analysis examines the relationships between columns.
- Cross-table profiling
With this technique, multiple columns across different tables are analyzed to locate broader relationships and dependencies. Cross-table profiling often brings about stray data and discrepancies.
Alternatively, you can leverage an AI-powered large language model (LLM) to automate this process. LLMs can streamline data profiling by identifying patterns and anomalies in your data. As a result, you can focus on high-priority tasks and make data-driven decisions with confidence.
3. Establish Consistency through Data Standardization
It is essential to use consistent data formats, naming conventions, and values across all systems and departments. This way, everyone can interpret and use the data accurately without confusion or errors.
Data standardization makes this possible. With data standardization, you convert the structure of datasets into one common format.
To ensure data standardization, just define the formats for various types of data within your organization. For example, decide whether to use “DD/MM/YYYY” or “MM/DD/YYYY” for dates. Then, create rules that ensure only data meeting these formats can be entered into your systems.
Data standardization promotes better internal communication and more reliable reporting, which leads to more accurate data-driven decision-making.
4. Clean Up Your Data
When you deal with large amounts of data, duplicate records and missing values are bound to appear. If not addressed, these issues can skew analysis and lead to incorrect conclusions.
As a result, it’s essential to clean your data. Data cleansing happens after profiling and standardization. It is the process of fixing (or removing) corrupt, incorrectly formatted, duplicate, or incomplete data discovered during the profiling phase.
You can equally optimize this phase by using data-cleaning software. This solution can automatically detect and fix common data quality issues while maintaining a detailed audit trail of all changes made.
5. Validate and Verify for Reliable Results
Now, you need to validate and verify your data. With data validation and verification, you ensure your final data actually adds value to your organization.
Data validation is the process of determining whether a specific piece of information falls within its acceptable range of values. For instance, during data validation, you might check if all the US phone numbers in your dataset have ten digits, not more, not less.
Data validation should be easy if you set up automation rules that check for anomalies and discrepancies in real-time. We already talked about how you should create such rules to flag date formats that don’t meet your organization’s format standards as part of data standardization. You should do the same to validate other important data such as email address syntaxes and numeric values.
If you’re handling customer information, automated validations can instantly flag entries where email addresses are missing the “@” symbol or phone numbers are missing a digit. This instant feedback ensures quick corrections can be made before further processing.
Data verification, meanwhile, is the process of checking the accuracy of the data per se.
Depending on the nature of the piece of information, you can perform data verification by going straight to the original source or by using tools.
For instance, to determine if an address entry is actually your customer’s address, you can just send them an email and ask them to make the confirmation or adjustment.
But if you want to determine the accuracy of an address entry that says Helsinki is in Finland, you’d need to use tools with an AI framework like retrieval-augmented generation (RAG). What is RAG architecture ? It’s a system that enhances the output of large language models by pulling in external data from a knowledge base. So, when you ask an AI tool where Helsinki is, it can give you the right answer.
6. Connect the Dots with Seamless Data Integration
Data integration is the process of combining data from multiple sources into a unified view. It’s a step that occurs after you’ve standardized your data, cleaned it up, and validated and verified it.
The first step to integrating data is identifying key data sources. This could include customer relationship management (CRM) data, marketing automation platform data, or enterprise resource planning (ERP) data.
Next, map the data fields. Align similar fields from different sources to ensure information is stored in a consistent manner. For instance, you can map customer names from your CRM data to customer names in your ERP data, ensuring a unified view of customer information.
Finally, employ extract, transform, and load (ETL) tools to transfer the data into a central repository. This can be a data warehouse or a unified data platform.
By doing so, you ensure data is accessible and usable across the organization, enabling more accurate reporting.
7. Secure Your Data
If you want to preserve the integrity of your data, you must secure it. So, comply with applicable privacy legislation and implement procedures to prevent customer data from being accessed without authorization.
Also, implement robust security measures, such as data encryption , access controls, and regular backups to prevent data breaches and losses.
When you secure your data, you don’t just ensure its integrity. You also ensure its confidentiality and availability.
8. Monitor and Adjust for Continuous Data Excellence
Data quality assurance is not a one-time task. It’s a continuous process.
You need to regularly inspect your data, identify areas for improvement, and make adjustments to prevent data quality issues from creeping back in.
To achieve this, set up a data quality dashboard that provides real-time insights into data health and performance. This dashboard can track key metrics such as data completeness, accuracy, and freshness.
Review these metrics to detect anomalies or trends indicating data quality issues. If you find any, take the necessary corrective steps.
Let’s say you notice a sudden spike in duplicate records. If you find the duplicate entries are caused by a glitch in your data entry platform, you might decide to replace your tool or call your provider’s customer support.
Regularly monitoring and updating your data makes you confident in its accuracy and reliability. In turn, this leads to better decision-making and a competitive edge.
Conclusion
Data quality assurance ensures you make critical business decisions based on accurate information. Doing so leads to positive outcomes like increased profitability, improved operational efficiency, and enhanced customer satisfaction. Follow the steps above to ensure robust data quality assurance for your business and reap its benefits.
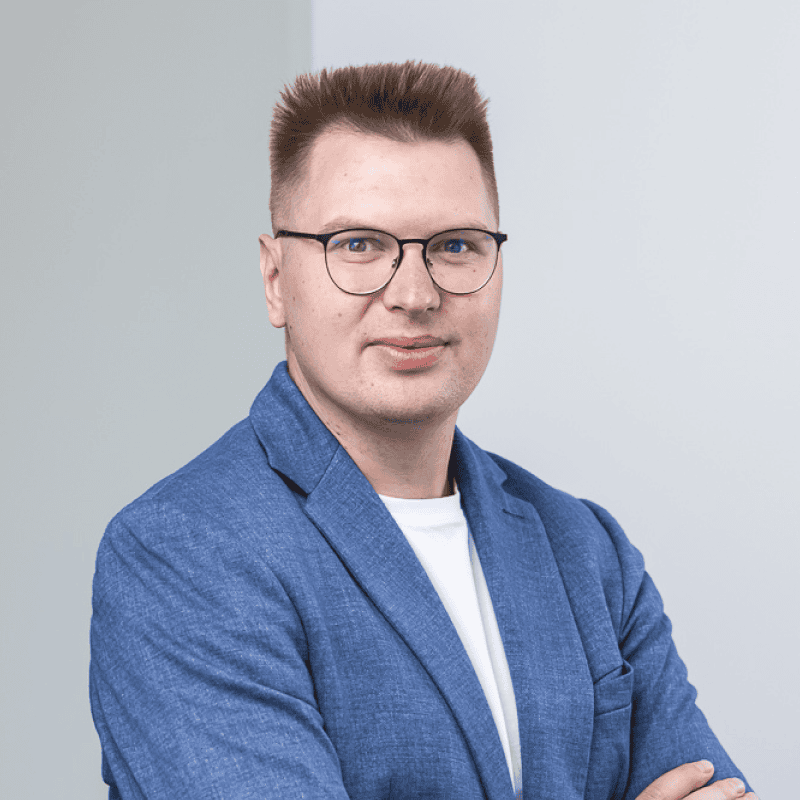
Author
Justas Vitaitis
Senior Software Engineer
Justas is a Senior Software Engineer with over a decade of proven expertise. He currently holds a crucial role in IPRoyal’s development team, regularly demonstrating his profound expertise in the Go programming language, contributing significantly to the company’s technological evolution. Justas is pivotal in maintaining our proxy network, serving as the authority on all aspects of proxies. Beyond coding, Justas is a passionate travel enthusiast and automotive aficionado, seamlessly blending his tech finesse with a passion for exploration.
Learn More About Justas Vitaitis