What Is the Cost of Poor Data Quality?
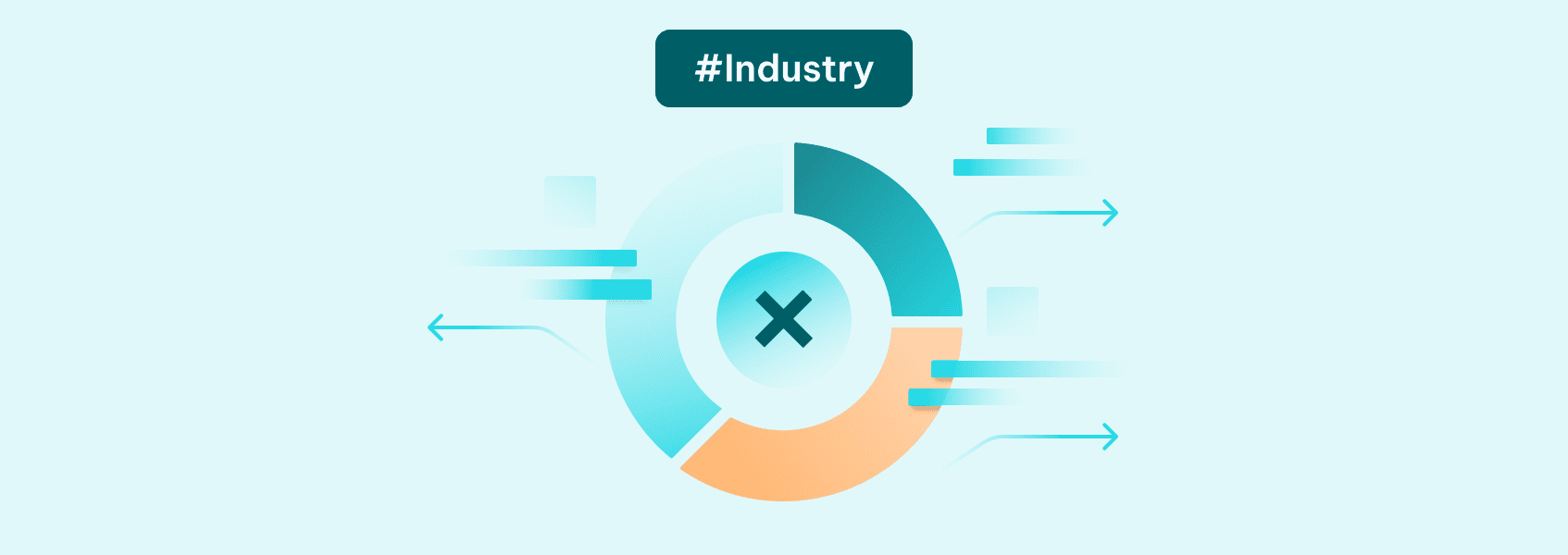
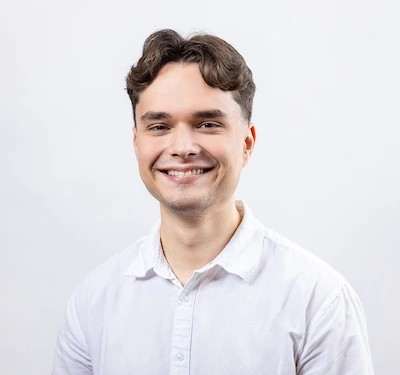
Justas Palekas
In This Article
Many digital businesses now consider themselves to be data-driven. The ubiquity and ease of collection through various software solutions has enabled companies to collect tons of data passively and implement it in their decision-making.
Yet, it’s often too easy to look at dashboards and tools without considering the data quality. Gartner estimates that poor-quality data costs businesses an average of $12.9 million per year.
The Real Cost of Poor Data Quality
Data quality can be difficult to evaluate as it’s simply information about the real world. There’s no underlying principle that would show that the information stored in systems is inaccurate data with the exception of missing or nil fields.
As such, it’s easy to convince yourself that the data shown in a dashboard is accurate and of high quality. If it is then used to make conclusions, these decisions are based on an incomplete picture. Decisions based on poor-quality data can lead businesses to focus on the wrong products or services, leading to a loss of revenue.
Additionally, it can be difficult to discover that decisions were based on poor-quality data. Most decisions and strategies take time to implement, and results may come even later. Due to the long delay between decisions and results, poor-quality data may be affecting a significant number of business operations.
There are also indirect bad data effects. Since decision-making can lead businesses to focus on one area and revenue stream, there will always be lost opportunities along the way. These lost opportunities may have been significantly more profitable or viable than anything that was based on bad data.
In some cases, poor data quality can also lead to reputational or morale damage within the company. Bad decisions made through inaccurate data will erode trust in both top-level management and employees, leading to overall worse performance in the long run.
Understanding Data Quality
Data quality is a difficult concept that’s constantly being researched by both academics and businesses. While there’s no singular definition, most researchers define two categories of data quality metrics – intrinsic and extrinsic.
Intrinsic metrics are those that can be validated without reference to use cases or practical application. For example, common data errors are missing fields, issues with value or entity uniqueness, and duplicate entries.
All of these data quality issues can be resolved through warehouse or source management. Engineers and other team members can implement various strategies and data validation tools to resolve these issues.
Some commonly defined intrinsic data quality metrics are:
- Accuracy – how well data describes the world
- Completeness – how complete is the model and how many possible fields have appropriate values
- Consistency – whether entities and values are properly assigned
- Timeliness – how fresh is the data.
Timeliness is sometimes also put into the extrinsic data quality metric category. While it can be evaluated purely on timestamps and recency, the importance of recency will often be defined by practical use.
On the other hand, extrinsic data quality metrics are those that are directly related to the business use case. Poor data quality of this type can only be resolved by collaboration between analysts and stakeholders such as C-level executives.
Some commonly defined extrinsic data quality metrics are:
- Relevance – whether the data provided is suitable for the use case at hand
- Reliability – whether data integrity has been maintained and the information is trustworthy
- Usability – whether the data provided is easy to understand and use.
Each of these data quality dimensions are essential to maintaining trust and effectiveness in decision making processes. Extrinsic factors are often the first to be targeted for management as the inability to make use of data, no matter how consistent and complete, has little practical value to a business.
What Causes Poor Data Quality?
Poor data quality can be caused by a wide variety of factors, ranging from human error to technical difficulties of different kinds. Often, businesses with issues related to bad data will have several processes taking part at once, making it harder to discover the most pressing reason.
Human Error
Human error is the simplest and one of the most common reasons for bad data. Even if little work is performed manually, human error can happen at any stage of the data quality management process.
Most errors happen at the data entry stage. These errors can scale quite quickly with the amount of manual work that must be performed. As such, manual data entry should be minimized wherever possible.
Additionally, human error can happen at other stages of the process, such as when data is being transformed, moved, copied, or reformatted. These errors, however, are usually easier to notice as larger parts of data sets are affected.
Lack of Data Standardization
Data scientists and engineers will often speak of the importance of standardization. A common example of poor standardization is when a database may be using different ways to represent the same information (such as using “USA”, “US”, and “United States of America” in the same set).
Lack of standardization causes poor data quality by duplicating entries. In large sets, quantitative analysis for “United States of America” might return incorrect data as it would miss the other notations (“USA”, “US”).
Luckily, improving data quality in this regard is relatively easy for smaller businesses. Standardizing sets of information and entity IDs or names will significantly reduce the likelihood of bad data. For larger businesses and enterprises, data governance strategies will be required.
Poor Data Governance
Data governance is the practice of managing information within a company by implementing best practices and processes. In large organizations, data scientists and engineers are only a small part of people directly involved with managing information.
As the number of stakeholders rises, especially people outside of the field of data quality management, the likelihood of various errors rises as well. These may include, but are not limited to data entry, transformation, or inconsistent update issues.
Lackluster Data Integration
Maintaining high-quality data in larger enterprises means collecting information from a wide variety of sources. Most of these sources will use different notations and formats, necessitating various processes to maintain good quality data.
These issues may be relatively minor if data is being loaded from automated internal sources. Whenever manually entered information such as customer data is included, the issues may become more pressing as there may be a significant increase in errors.
Finally, external sources (such as through web scraping) may cause data integrity issues. Most of such data will be unstructured, which will require significant transformation efforts. Even with the best intentions, data scientists and analysts will have to take extreme care when integrating such information.
How to Improve Data Quality?
Good data quality is a matter of definition. Few businesses, if any, can maintain high-quality data at all times and at every point in the collection and analysis process. As such, it’s often recommended to start from the extrinsic data quality metrics.
Improving data quality, as such, starts with defining a use case for the data. Currently, common examples include the development of machine learning or AI models, developing business strategies, and optimizing resource management.
Once a use case has been defined, stakeholders can discuss all of the data quality issues. For example, is bad data causing machine learning models to fail accuracy benchmarks, or is improperly managed customer data causing stakeholders to lose efficiency when devising sales strategies?
These issues will usually point towards an intrinsic data quality metric. In the example of customer data, there may be accuracy or completeness issues, which is causing the underlying problem. Additional data validation steps could resolve the issues completely.
Such a process helps companies pick out the intrinsic data quality metrics to focus on. Sometimes, however, intrinsic data quality metrics may not be at fault. Depending on the issue, extrinsic data quality metrics could be at fault as well.
For example, if users are constantly asking for clarification in regard to interpretation, there may be no need to improve data quality itself. Data scientists may be presenting the end results of their work in a muddled fashion, making it harder for non-technical users to understand it.
Conclusion
Bad data can be the culprit of many organizational issues, ranging from simple errors to major losses in revenue. Maintaining good quality data is not only essential to effective decision-making but in preserving trust in data itself.
While bad data can be a significant burden on a company, good-quality data can bring tremendous benefits. There’s one pitfall many organizations fall into – treating data as a good in itself that requires no maintenance. It’s an asset like any other, as data can depreciate in value and become useless. In some cases, it may even become harmful, therefore, great care needs to be taken to manage it.
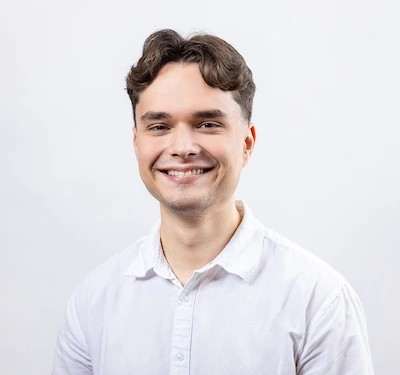
Author
Justas Palekas
Head of Product
Since day one, Justas has been essential in defining the way IPRoyal presents itself to the world. His experience in the proxy and marketing industry enabled IPRoyal to stay at the forefront of innovation, actively shaping the proxy business landscape. Justas focuses on developing and fine-tuning marketing strategies, attending industry-related events, and studying user behavior to ensure the best experience for IPRoyal clients worldwide. Outside of work, you’ll find him exploring the complexities of human behavior or delving into the startup ecosystem.
Learn More About Justas Palekas