Data Quality Metrics: How to Measure the Accuracy of Your Data
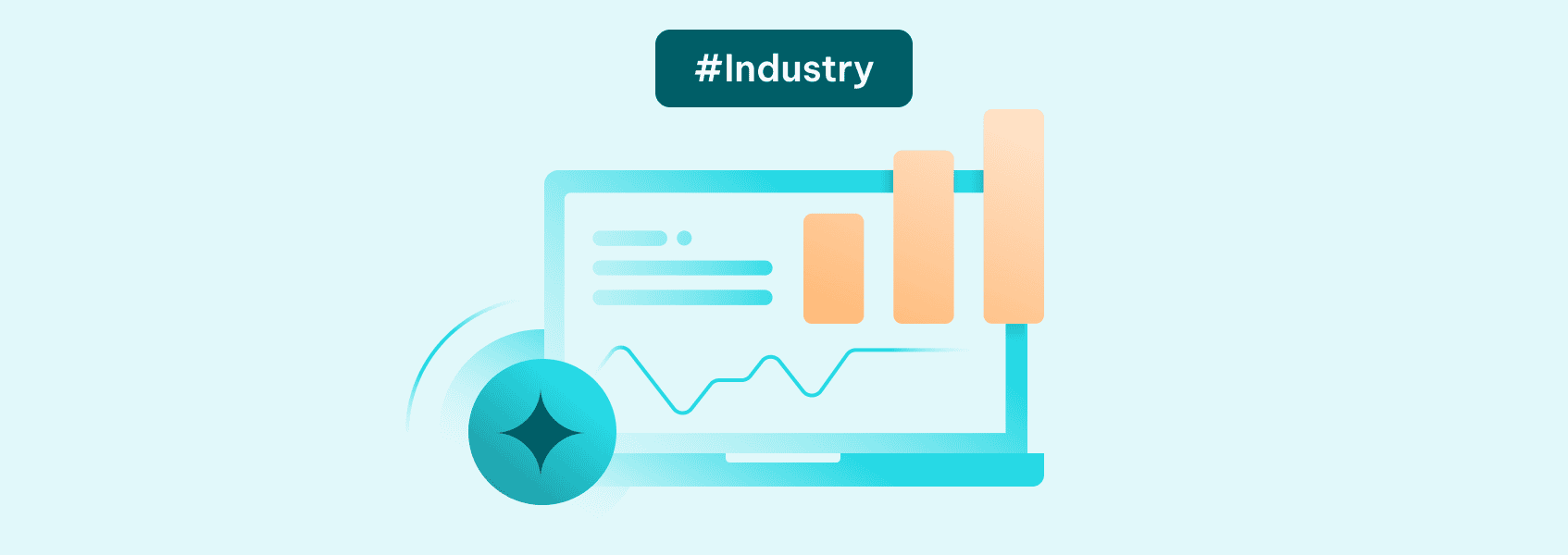
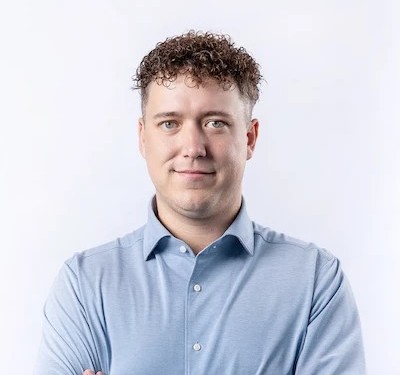
Vilius Dumcius
In This Article
Data quality should be an essential part of any data-driven operations. Even for businesses that don’t intend to sell datasets to other companies, the quality and accuracy of data will greatly influence decision-making effectiveness.
Unfortunately, there’s no single metric you can track to make sure data quality is up to par. You’ll have to track several metrics and constantly keep an eye on them. As such, maintaining data quality is a constant process that requires time and effort.
What Is Data Quality?
Data quality is a general-purpose term that refers to the usability of information for its intended purpose. A simple example of data quality is the accounting information of a business. If you look at a monthly revenue-costs report, does it accurately reflect the actual spending and money inflows?
Poor data quality can have a tremendous impact on overall business performance and decision-making. In the case of an inaccurate revenue-costs report, the business may be either spending too much money or improperly reinvesting profits.
Similar cases can arise in other areas and cause decision-makers to focus on the wrong products, marketing efforts, etc. Data quality metrics, as such, are essential to maintaining trust and confidence in both the sources of information and decision-makers.
On the other hand, top-notch data quality will provide a foundation for effective organizational actions. Decision-makers can much more easily select marketing campaigns and winning products, which may continue to raise business profitability.
What Are The Data Quality Metrics?
Most data integrity and quality researchers define two categories of data quality metrics (sometimes also called dimensions) – intrinsic and extrinsic.
Intrinsic data quality metrics measure internal factors such as accuracy, completeness, consistency, etc. Extrinsic data quality metrics measure how well the information fits the real world through aspects such as timeliness, relevance, reliability, usability, etc.
Both categories are essential to high-quality data. Without intrinsic metrics, data may be hard to analyze, and hypotheses may be hard to test or validate. Without extrinsic metrics, the data may be hard to adapt to real-world conditions and decisions.
Intrinsic data quality dimensions are often managed and taken care of within the collection or analysis team. Factors such as accuracy and completeness of data values are completely independent of any actual use case. In other terms, these are purely analytical concepts.
As such, data quality controls have to be implemented in the early stages of any collection practice. Managing data sources and verifying that you’ve received accurate information, for example, is one control component.
Additionally, data engineers should be employed to manage the data warehouse and normalize and clean up information. Warehouses will usually extract data from numerous internal and external sources where everything may be stored differently, ranging from formatting to completely unstructured information.
Extrinsic data quality dimensions are managed on the other end of the business – stakeholders. They should be able to clearly and accurately define use cases in order to avoid inefficient workloads and usage of redundant data. While they have little to no influence on improving data quality, stakeholders have to ensure that information is being utilized appropriately.
Types of Data Quality Metrics
There are numerous data quality dimensions that can be improved upon. While high-quality data would ideally manage all of them, sometimes an organization is capable of only focusing on improving on several of them at a time. Picking the correct data quality dimensions becomes vital to data quality assessment.
Intrinsic
Intrinsic metrics measure the quality of data based on inherent characteristics, focusing on data content and structure.
Accuracy
Data accuracy measures how well the collected information describes the real world. For example, an invoice is a data source that describes services rendered, the date of provision, and the payment made. If any of these data points are incorrect, then the data accuracy is off.
It should be noted, however, that data accuracy is fractional. If only the date on the invoice is incorrect, the invoice is still a valuable source of data. To improve data accuracy, create a reference set, verify it through other people, or check it against rules that prevent data errors.
Completeness
Data completeness defines the totality of the description instead of the accuracy. A single invoice will not describe the entirety of a business’s revenue and costs, but everything in the accounting system might.
Issues with completeness can be uncovered by looking for missing fields or data points. It may also be validated by looking at input mechanisms and measuring whether the description provided is satisfactory.
Consistency
Data consistency measures whether values and data points are internally consistent. In cases where there are redundant data points, it may be validated by looking at whether the values are identical.
Consistency metrics are usually tied to the uniqueness of either values or entities in the data set. Additionally, data quality in terms of consistency may be checked through various methods, such as referential data integrity checks.
Privacy and Security
Both of these data quality dimensions are defined by various laws and regulations. Additionally, certain data types (such as medical or personal data) will have more stringent compliance requirements.
Privacy and security data quality metrics are measured by verifying access controls. Usually, these are how many people have access to data storage and the amount of unmasked information.
Timeliness
Data timeliness is defined by the freshness of information. While always being up-to-date is preferable, different requirements may be upheld.
Timeliness may be measured by these data metrics: the comparison of timestamps to the current moment, the last update when compared to the expected rate of change, and the corroboration of other data points.
It’s also one of the few data quality metrics that also belongs to the extrinsic category, as it may be measured by how well it fits a use case’s requirements.
Extrinsic
Extrinsic data quality metrics are task or use-case dependent. Stakeholders will usually tell analysts whether the data attributes are up to par.
Relevance
Out of all the extrinsic data quality metrics, relevance is the most important. Put simply, it’s the question of whether the data presented is suitable for the required task.
Tests for this data quality assessment can range from qualitative questions (such as asking whether there’s enough information to complete the task), checking how many ad hoc data lookups are happening, and how many questions are sent to various support personnel.
Reliability
Similarly to data integrity, reliability is a data quality measurement that defines trust and credibility in sources and resource management. Good data quality metrics for reliability are how easy it is to verify, whether there’s enough information about lineage, and if bias has been minimized.
It can also be tracked by checking how many users attempt to access the source of the data and how many users appear or are newly created when a new project is undertaken.
Usability
Usability defines how easy it is to access and review data. For example, dashboards with high data integrity and clarity would have good usability. If there are data errors, ambiguity, or difficulties interpreting, that indicates low usability.
As one of the more practical data quality metrics, most of the verification happens through qualitative processes. These may be requests to present data differently, requests to provide help interpreting, etc.
How to Get Started With The Right Data Quality Metrics
Few businesses have the resources necessary to start implementing all of the above data quality measures. If we add some of the less often mentioned data quality metrics (validity, sufficiency, bias, conciseness, etc.), it’s likely that all businesses would have to choose some to start taking care of.
While intrinsic data quality metrics have the benefit of being managed by a smaller team as there are no stakeholders involved, they’re mostly used for clarity, optimization, and security purposes.
As such, it’s often best to start with use cases and practical applications of data. If a company collects and manages large amounts of data, it’s likely they have some practical application for it.
Before heading off to implement data quality standards, you should first consider which of the applications are most useful and work towards improving their performance.
Once you have the use cases in place, look for the issues stakeholders raise most frequently. These data quality issues will serve as guidance for potential areas of improvement. For example, low-quality data may force users to constantly validate information manually.
Issues raised will have a direct connection to data quality metrics. For example, the constant need to validate information indicates data quality issues in the accuracy and completeness areas. Inconsistent data values, on the other hand, indicate issues with internal consistency.
Once these areas are identified, it’s important to establish ways to measure data quality improvements. For example, if users were constantly validating information by accessing the warehouse themselves, a reduction in such actions indicates an improvement.
Conclusion
Data quality is the lifeblood of any organization that uses information to support decision-making. Poor data quality can cause inaccurate conclusions, improper strategy formation, or even revenue loss.
While some people might think that low quality means inconsistent and mismanaged data values, the process goes much further than that. Managing data quality means working closely with various departments to ensure that information is trustworthy, clear, and concise.
Data quality management goes far beyond what we’ve mentioned in the article. There are many more metrics, strategies, and processes to keep in mind. The entire field of data quality management is immense, studied by both businesses and academics alike. Constant improvement and monitoring, however, will make any data quality practices much more effective.
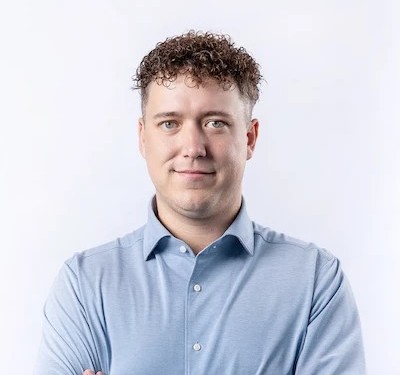
Author
Vilius Dumcius
Product Owner
With six years of programming experience, Vilius specializes in full-stack web development with PHP (Laravel), MySQL, Docker, Vue.js, and Typescript. Managing a skilled team at IPRoyal for years, he excels in overseeing diverse web projects and custom solutions. Vilius plays a critical role in managing proxy-related tasks for the company, serving as the lead programmer involved in every aspect of the business. Outside of his professional duties, Vilius channels his passion for personal and professional growth, balancing his tech expertise with a commitment to continuous improvement.
Learn More About Vilius Dumcius