Understanding Data as a Service (DaaS) and Its Significance
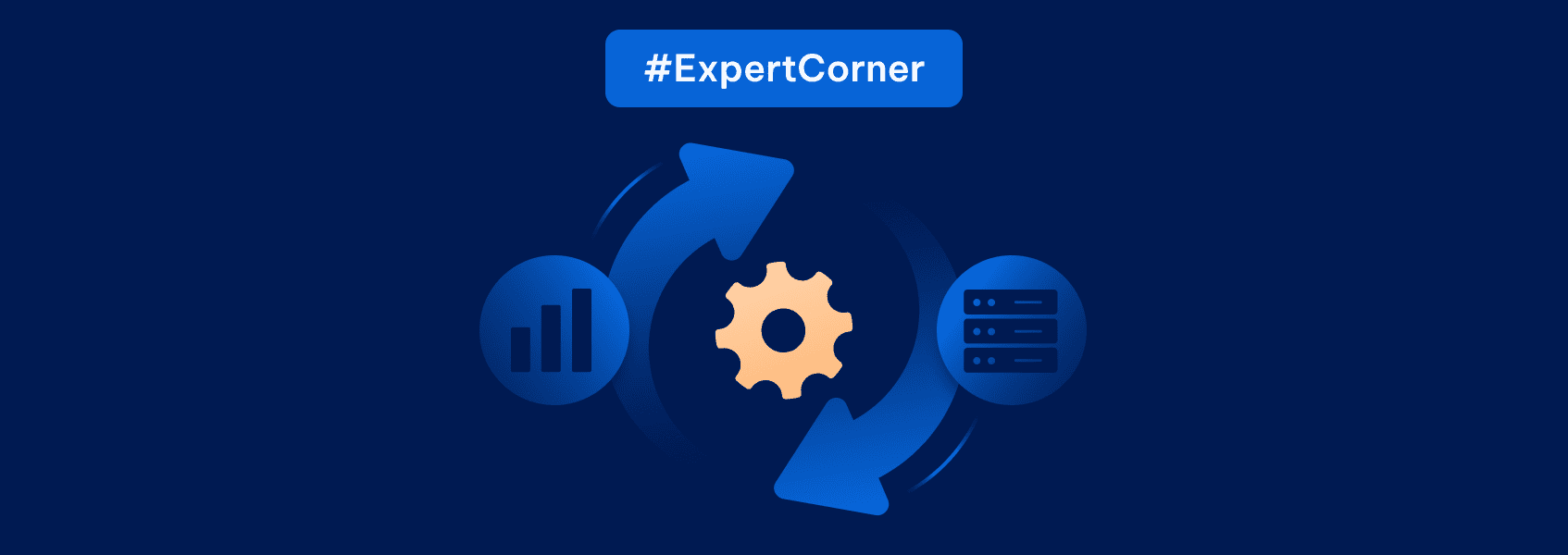
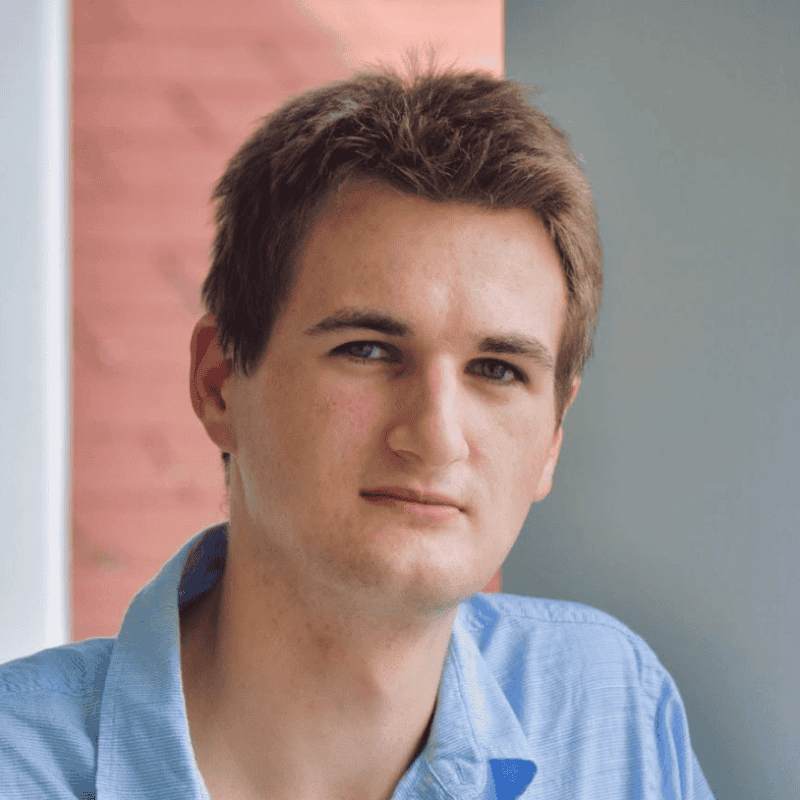
Zach Quinn
In This Article
If you work in the data and business intelligence space or work closely with teams linked to data infrastructure, you’ve likely heard of cloud computing platforms as a service (aaS) offerings. You’ve probably heard acronyms ranging from platform as a service (PaaS) to software as a service (SaaS). Now you have one more aaS acronym to add to your big data pocket dictionary: DaaS.
Data as a service (DaaS) is an on-demand business model wherein a company has access to data of varying quality and types, including both structured data (think spreadsheets) and unstructured data (think device metadata and IoT streaming logs). Before providing data to client organizations, DaaS businesses perform data preparation steps, including programmatic data cleaning, to ensure clients receive clean data to provide to their data teams and leadership.
To get the data into the hands of end users, DaaS businesses leverage cloud technology to deliver data wirelessly and without either organization needing to worry about on-prem infrastructure. If it’s helpful, think of this transaction as an API subscription, where customers can control the volume and granularity of data they receive.
How Do Data as a Service Business Work?
The first step of any DaaS process is data acquisition. One of the most popular sayings in the field of big data is that data is the new oil . And just like rigs drilling for oil, DaaS companies have a wealth of sources that provide this valuable raw resource. Typically, DaaS companies begin with structured data stored in publicly accessible repositories, like government records or university databases.
However, what really distinguishes any DaaS operation is not its selection of public structured data but its capacity to mine and refine unstructured data. A DaaS company’s most significant competitive advantage is its ability to efficiently and accurately convert this extraneous data to a human-readable format.
Data as a service business model leverages two methods, cloud computing and web scraping, to fulfill two distinct steps in the DaaS life cycle: Data acquisition and data provision.
Cloud computing provides data as a service businesses with the flexibility to provide customers access to datasets or files directly through serverless repositories or even by setting up data pipelines to stream data directly to customers.
For example, a data as a service business may provide subscription-based pricing with a flat monthly fee. Many of these businesses, however, will use per-line or per-data-point pricing. From a user perspective, customers can access the database to download specific information whenever they want but ultimately pay a fee based on how much data they retrieve in a given billing period.
Cloud computing provides both parties with incredible flexibility, and cloud offerings allow data as a service businesses to benefit from increased market size as they reach international clients. Since data legislation is still in its infancy, they can now sell their data to nearly any company in the world with few regulatory or infrastructure barriers.
Web scraping is another technology that revolutionized data as a service. Instead of being able to procure data from partners and agencies, web scraping allows businesses to collect data directly from nearly any page on the Internet. Using a program developed by a software engineer or data engineer, DaaS businesses can build applications that can scan millions of web pages, parse the data and create diverse, accessible databases bursting with valuable information.
Although data gleaned from web scraping is often unstructured and “messy” at first glance, what it lacks in form it makes up for in timeliness, allowing companies to ingest real-time insights by monitoring end user and competitor behavior on highly trafficked sites like various social media channels.
Advantages of Data as a Service
Choosing to work with a DaaS provider creates distinct benefits for companies that can handle and analyze information internally. However, note that DaaS businesses aren’t as useful to those that don’t have internal data analytics teams , as very few organizations can parse and understand data without the proper experts employed and infrastructure in place.
Cost Reduction
Data as a service businesses can collect and store data at a significant scale, which allows them to improve unit economics and get better deals when partnering with SaaS and PaaS cloud vendors. Therefore, using a DaaS provider will often be cheaper than procuring the same data on your own.
Additionally, using a DaaS provider reduces the scope of data and software roles necessary to procure data, especially since tasks like developing and deploying web scraping scripts can be complex, timely endeavors.
Flexible Scalability
Through a DaaS provider, businesses can quickly scale or reduce their data analysis and business operations. When data storage and extraction are handled in-house, some of the talent and assets may go to waste when scaling down. On the other hand, scaling up is usually significantly slower if new people need to be hired.
DaaS businesses instead provide data access that’s easily customizable according to a client’s current needs. Consequently, DaaS providers enable companies to handle large volumes of data more seamlessly without needing to create infrastructure from scratch.
High Data Quality
While customers usually have no control over data quality, data as a service businesses perceive data quality to be an important competitive advantage. As such, as a DaaS provider scales ingestion infrastructure, they make sure not to do so at the expense of data accuracy .
Challenges in DaaS Implementation
While DaaS can improve business practices and decision-making, it’s often not as easy as purchasing any other service or product. Big data is not an out-of-the-box resource. Data solutions need to be implemented and integrated into existing warehouses and be transformed to provide data-driven insights and analytics to decision makers.
Data Security and Compliance
As data privacy legislation gains traction around the globe , it is important for all parties in a DaaS transaction to understand that most data is at least somewhat sensitive, with some forms of it being extremely carefully monitored for any misuse (such as personal or health data). The most sensitive information is known as personally identifiable information (PII) , which might include anything from credit card information to social security numbers.
As such, both parties need to be extremely cautious when procuring or acquiring data. Data access has to be restricted and carefully monitored by the proper data governance professionals to avoid leaks on the part of both DaaS providers and end users.
These challenges are best overcome by properly selecting data services themselves. Providers should have implemented various security measures, possess certifications, and implement strict risk and legal management procedures to ensure that everything operates within appropriate legal boundaries.
This is best accomplished by conceiving and implementing a robust and comprehensive data management strategy, as the field is constantly evolving with new data privacy risks presenting themselves on a daily basis .
Technical Data Integration
Data as a service providers may store their information in various file formats and management systems, which won’t always seamlessly integrate with a customer’s existing data infrastructure or tech stack. Data assets may need to be converted into different formats in order to be properly and accurately ingested into a data warehouse. Even tasks that might seem mundane, like adding a column or modifying a data type, can take time.
Additionally, managing several sources of data assets is always more difficult than handling everything in-house . Customers purchasing data services should always discuss the integration process and try to find ways that work for both companies.
DaaS Use Cases
Thanks to its versatile approach, the DaaS model can be applied across various industries and business functions. Some of the most common ones include:
Ecommerce
Online retail, a.k.a. ecommerce, can benefit from access to expansive customer datasets they would have difficulty accessing otherwise. Since the industry is extremely competitive with paper-thin margins, data is often the way to create or maintain a competitive advantage.
Third-party data sources can help ecommerce companies create novel business strategies, enhance predictive analytics, or find secondary signals that indicate changing consumer behaviors.
Customer Relationship Management and Sales
While customer relationship management (CRM) data arrives through internal channels, it can be heavily enhanced with DaaS services. One easy way to implement such enhancements is to enrich CRM data assets with third-party sources to update customer and business profiles using publicly available information like a customer’s email, job title, or industry.
Automated data analysis can also be used to segment customers into various profiles and bucketing them into categories depending on their likelihood to purchase a particular good or service or to purchase a recurring subscription.
Machine Learning
Businesses that train and deploy machine learning models can uniquely benefit from a DaaS company’s offerings. Data a DaaS business acquires and provides can satiate even the “hungriest” big data ML model.
Since ML models are prone to consuming “junk” data , data quality controls have to be extremely strict to create accurate and valuable predictive models. Data as a service companies are in a position to provide machine learning developers with both assets and consultation on data management which, in turn, can provide more accurate, tailored data to enhance the accuracy and precision of ML models.
SaaS vs. DaaS
Software as a service (SaaS) has been one of the most prolific and rapidly growing industries in the world, approaching a market cap of 1.2 trillion by 2032 . Incidentally, there is a lot of overlap between SaaS and DaaS, especially with regard to how the product is provided to end users.
Both business models use cloud technologies to provide customers all over the world with frictionless access to their products. SaaS vendors, however, provide various software solutions (such as communication or data management tools) to businesses and consumers.
On the other hand, data as a service businesses provide access to data assets. While they use data management software to maintain vast troves of information, the companies don’t sell those solutions. In fact, they may often use infrastructure provided by a SaaS business, like a remote server, to store and make accessible the data they offer to customers.
Additionally, data as a service companies exist at a different part of the value chain . They may even collect data from various software-based sources to enhance their business operations themselves. In some cases, such data may also be sold to third parties, although that’s subject to many caveats and legal protections.
As such, SaaS and DaaS use the same methods to sell their products, but the products themselves are entirely different. These two sectors, however, are ultimately symbiotic.
Without SaaS, DaaS would be difficult to scale and maintain.
The Influence of DaaS
While data as a service is still a relatively new business model, it has incredible potential to revolutionize many aspects of decision-making. Decision makers can devise a new data management strategy, enhance existing products and services, make use of advanced analytics for business expansion and further refine competitive technologies like in-house ML models.
Although it may seem like DaaS could reduce the need for data-oriented roles, DaaS businesses actually create jobs for roles at the data preparation stage of the data science lifecycle , specifically data engineers and analytics engineers.
Data-oriented roles provide competitive salaries which, in turn, can attract world-class talent to work to conceive and scale DaaS data infrastructure.
However, DaaS businesses do have a few vulnerabilities that need to be taken into account.
The biggest challenge of a DaaS-oriented business is that it is selling raw data, which can be an abstract product since data is only as valuable as action taken as a result of the insight provided. A common pitfall is procuring so much information that most of it sits unused .
Data governance strategies that handle proper ways to store, manage, and restrict access to information are necessary to maintain operational efficiency and ensure compliance at all times .
One of the largest challenges a DaaS business faces isn’t how it stores data but how it presents that data as a viable product. Since raw data isn’t aesthetically pleasing, it’s important to employ data visualization specialists to highlight key insights clients could glean from the data being sold.
With a current market size of over 300 billion USD and projected to grow to over 1 trillion USD by the end of the decade , the data and analytics market is likely to continue on a pace of rapid, positive growth, with DaaS businesses being well-positioned to service this explosive industry.
DaaS businesses stand to benefit considerably from continued reliance on consumer data to conduct traditional analysis and, more recently, to fuel large language AI models (LLMs).
So if you ever forget what DaaS stands for, don’t worry; you’ll be hearing about it for years to come.
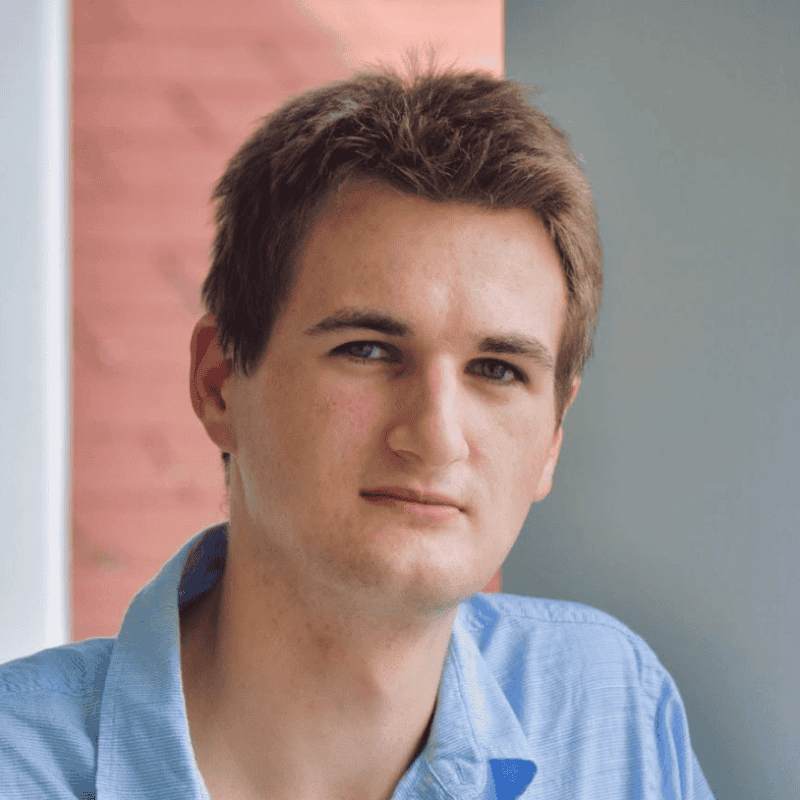
Author
Zach Quinn
Senior Data Engineer, Forbes
Zach Quinn is a senior data engineer within the media/publishing space. Holding an undergraduate degree in journalism and a master’s in data science, Zach specializes in making technical concepts compelling and digestible. He has founded and co-founded two Medium publications, respectively, Pipeline: Your Data Engineering Resource and Learning SQL, both of which have reached over 1 million readers by consistently publishing technical tips, career advice and engaging storytelling.
Learn More About Zach Quinn